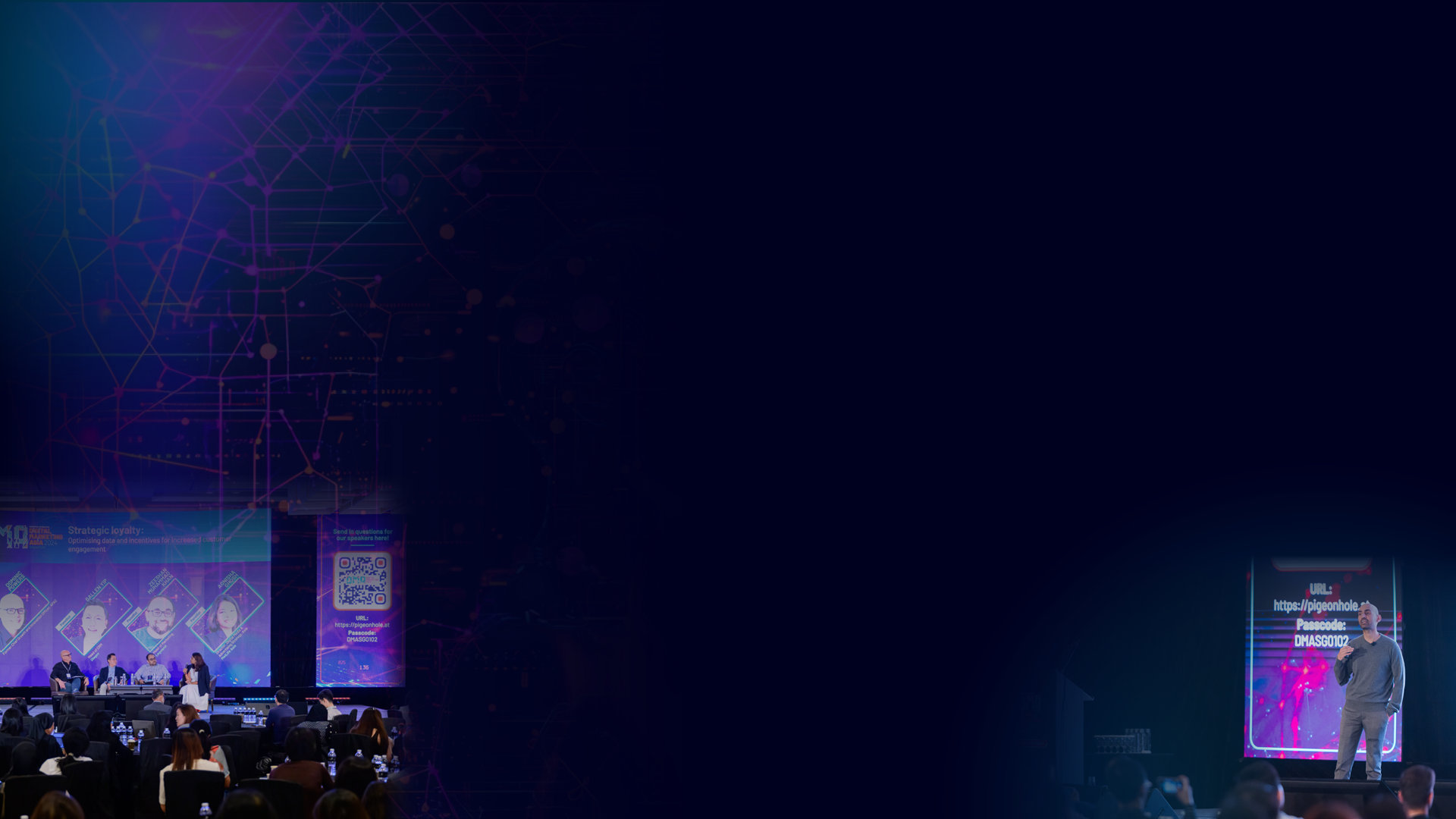
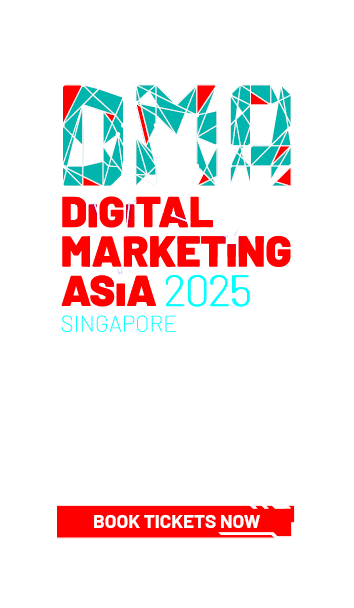
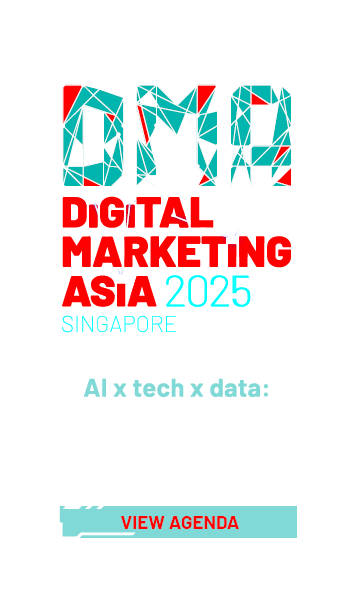
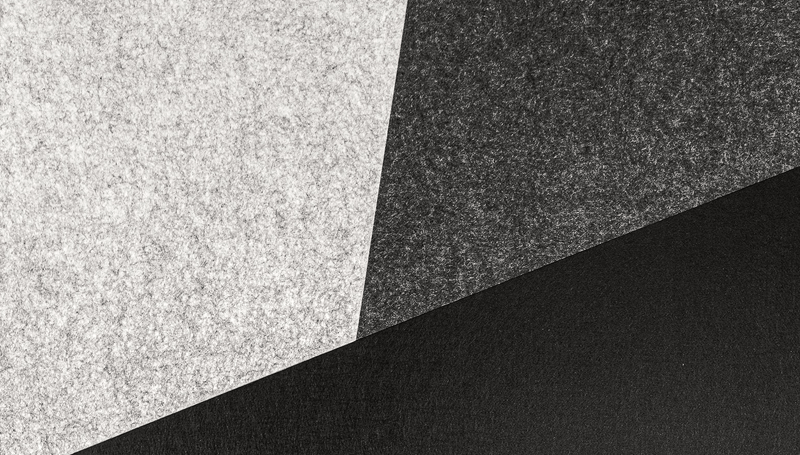
3 important steps of big data analytics you cannot miss
share on
Often do you hear the term “big data”, it has obviously become a hitting trend in nowadays business development and leads to the triumph. However, this can be quite challenging for you to execute it down-to-earth and how to kick-off. As a beginner, you must understand the 3 critical steps in a big data analytics project before you go further.1. Data preparationOne of the common challenges encountered by most businesses nowadays is lack of data for analysis. Even though they may accumulate membership or transaction data for years, those data are either in inconsistent format, outdated, invalid, or distributed in different systems or under different departments’ control. It usually consumes a lot of time or cross-departmental effort to centralise all data, clean the lists and transform the data into a useful and machine-understandable format to computer before analysis happens, the step we called Data Engineering or Pre-Processing.Sometimes, in order to enrich the dataset for value-added service, Data Engineers can also develop crawlers (or bots) themselves to crawl additional data from the deepest of the external web pages available. For example, property agents can crawl the general crime rate (which is not officially announced by the Hong Kong Police Force) among 18 districts in Hong Kong from different online news media and provide better reference value to their prospects when selling houses.2. Data analyticsWhen all of the data are centralised, of good quality and in a consistent machine-readable format, it comes to the step of data analytics. Normally, we can define data analytics into descriptive analysis and predictive analysis. Descriptive Analysis uses business intelligence and data mining to find out the answer for “what has happened in the past?” The analysis findings are usually presented in a report or dashboard view, and it drills down data in order to uncover detail facts such as the cost of marketing, root cause of failures, key performance indicators, etc. In contrast, Predictive analysis aims to answer the question of “what could happen in the future?”by making use of different statistical models. The forecast result depends on finding out the significant hidden pattern or correlations among different datasets in the history. Statistical models will be built based on those pattern and correlations in the past and try to guess the probability of the same outcome that will happen in the future.For example, given the weather data for the past two years and if we discovered any correlation between it to the sales data of a retailer, we can predict the sales performance in the coming seven days with the weather forecast data. Under normal circumstances, more data we collected for analysis, higher accuracy it can be of the prediction. However, ultimately, it is a prediction and never 100% accuracy guarantee.3. Data visualisationAfter analysis, it is also crucial to display the results in an easy-to-understand way especially if you need to present it to the management. Data visualisation, a new term coming out in recent years, means the presentation of data in a graphical format that enables readers to grasp difficult concepts, identify patterns, tendency and correlations quickly and understand any insights easier. With the well-known data visualisation tool such as Tableau, QlikView or Microsoft Power BI, you can even drill down into different charts and graphs by adjusting different parameters in real-time and interactively changing what data combination you want to see and how it is processed. It definitely saves you a lot of time to create the same charts and graphs repeatedly just because of different parameter sets.In fact, “big data” is nothing new but just the more sophisticated use of data and changing it into real benefits to the business and society because of the advancement of computation power and cloud technology in the recent years. If you want to learn more about it, check out more details on http://www.radicasys.com/big-data-courseThe article is sponsored by Radica Systems limited and written by Irene Cheung, co-founder of Radica Systems limited.
share on
Follow us on our Telegram channel for the latest updates in the marketing and advertising scene.
Follow
Free newsletter
Get the daily lowdown on Asia's top marketing stories.
We break down the big and messy topics of the day so you're updated on the most important developments in Asia's marketing development – for free.
subscribe now open in new window